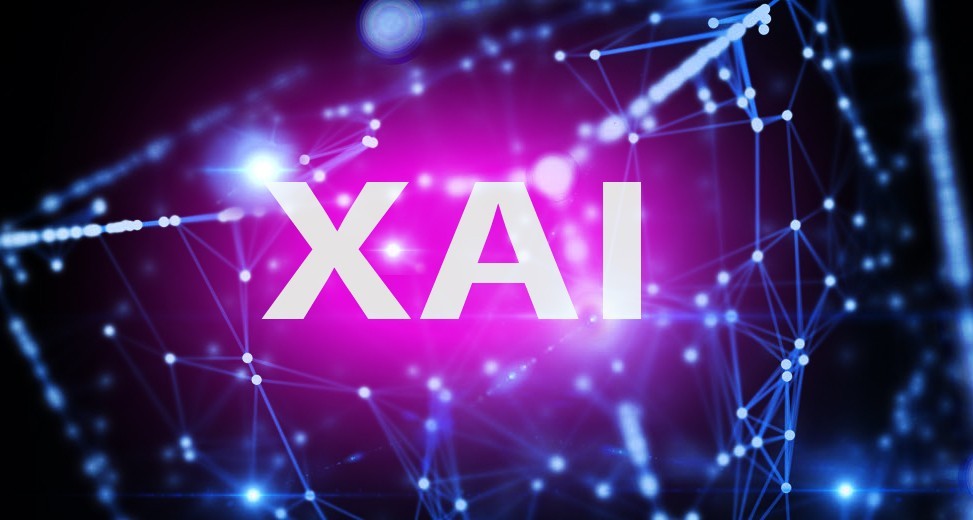
As artificial intelligence becomes deeply integrated into healthcare, finance, legal systems, and daily decision-making, a new concern has emerged: can we trust AI if we don’t understand how it works? This is where Explainable AI (XAI) comes in a growing field dedicated to making AI decisions transparent, interpretable, and accountable.
In this article, we explore what XAI is, why it’s essential, and how it strengthens ethical AI, especially through transparent AI models that prioritize fairness and trust.
What Is Explainable AI (XAI)?
Explainable AI (XAI) refers to AI systems designed to provide clear, understandable explanations of how decisions are made. Traditional machine learning models—especially deep learning systems—often function as “black boxes,” making decisions without revealing the reasoning behind them. XAI aims to open these black boxes and make AI decision-making more transparent and interpretable.
Key Characteristics of XAI:
- Interpretability: The ability for a human to understand why and how a model made a specific decision.
- Transparency: The model’s inner workings and logic are visible and understandable.
- Justifiability: The decisions can be justified with reasoning that aligns with human values.
Interpretable Machine Learning Models
While complex models like neural networks are accurate, they’re hard to interpret. XAI techniques apply to both intrinsically interpretable models (like decision trees or linear regression) and post-hoc interpretation methods for black-box models.
Common XAI Techniques:
- LIME (Local Interpretable Model-agnostic Explanations): Explains model predictions locally using simpler, understandable models.
- SHAP (SHapley Additive exPlanations): Assigns each feature a contribution value for a given prediction.
- Saliency Maps: Used in image classification to show which parts of an image contributed most to a decision.
- Model Distillation: Simplifies complex models into smaller, interpretable versions.
Why XAI Matters: Fairness, Accountability, and Trust
1. Fairness in AI Decisions
Without transparency, it’s difficult to identify biases that may be baked into training data or model logic. XAI helps expose and correct discriminatory patterns, ensuring decisions do not unfairly target individuals or groups.
2. Accountability in High-Stakes Applications
From loan approvals to medical diagnoses, automated decisions can significantly impact lives. XAI enables developers, regulators, and users to trace the reasoning behind each decision, making it possible to audit and appeal outcomes.
3. Building Public Trust in AI
Trust is earned when users understand and believe in the fairness and reliability of AI systems. Transparent AI models lead to higher user adoption and confidence, especially in sensitive domains like finance, legal, and public services.
Real-World Applications of Explainable AI
- Healthcare: XAI models help doctors understand AI-generated diagnoses and treatment recommendations.
- Finance: Transparent AI models are crucial for regulatory compliance and fraud detection.
- Hiring & HR: AI tools used in recruitment must explain why candidates are ranked or rejected to ensure fairness.
- Judicial Systems: Courts require AI-powered risk assessment tools to be interpretable and free from bias.
Challenges and Limitations
Despite its promise, XAI comes with challenges:
- Trade-offs with performance: Interpretable models may be less accurate than complex ones.
- Complexity of explanations: Some explanations are still too technical for non-experts.
- Lack of standardization: There is no universally accepted framework for XAI, complicating adoption.
The Future of Ethical and Transparent AI
As regulations like the EU AI Act and frameworks from the U.S. National Institute of Standards and Technology (NIST) emphasize transparency, organizations are being pushed toward adopting ethical AI practices that prioritize explainability.
Moving forward, Explainable AI will be essential for ensuring responsible innovation, user trust, and legal compliance in a world increasingly governed by algorithmic decision-making.
Conclusion
Explainable AI isn’t just a technical concept—it’s a moral imperative. By demanding transparency, interpretability, and accountability from AI systems, we can ensure that artificial intelligence serves all users fairly, ethically, and responsibly.